Exploring the Pros & Cons of GPU Cloud Servers for AI and ML
In the modern era of AI and ML, the requirement of powerful computing assets is now more meaningful as compared to previous years. Cutting-edge GPU servers, proficient at managing challenging tasks, that are necessary for training AI models, etc. Standard CPUs usually fail to fulfill the demands when it comes to performing parallel processing. This is the case where GPU cloud servers play a significant role, offering robust solutions for artificial intelligence and ML-based tasks. Let’s check out the pros and cons of utilizing GPU cloud hosting, with a complete focus on how NVIDIA GPU cloud services can boost your tasks.
About GPU Cloud Servers
GPU cloud servers are a type of virtual machine that are generally accelerated by Graphics Processing Units (GPUs) hosted especially on a specific cloud platform. These cloud servers provide the required computational power for heavy-computational tasks such as running AI models, and training ML algorithms. Cloud service providers such as GPU4HOST provide flexible solutions where every user can easily get the high-performance computing (HPC) power of GPUs without the requirement for physical setup.
Cloud-based GPUs allow organizations and individuals to boost cutting-edge GPU computing proficiencies without making significant ongoing investments in server infrastructure. With the potential of the NVIDIA GPU Cloud, artificial intelligence and ML-based tasks can get a good advantage from advanced performance, allowing quicker data processing, faster model training, and more productive scaling of assets.
Pros of GPU Cloud Servers for AI & ML
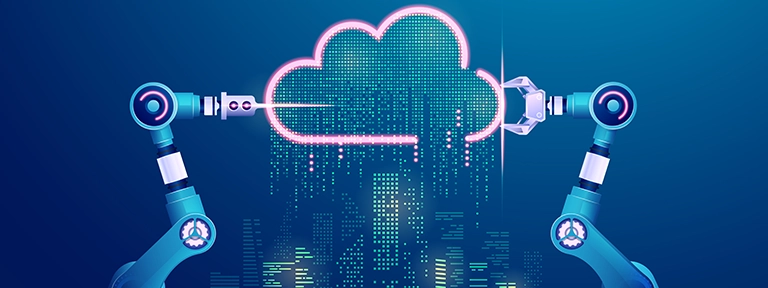
Budget-Friendliness
The key benefits of GPU cloud hosting is the proficiency to get advanced hardware without having the additional charges of buying, maintaining, and customizing physical servers. Standard GPU server setups need extensive capital investments, but with the help of GPU cloud services, anyone can effortlessly buy computing power according to their demands. This easy-to-pay pricing model makes GPU cloud services a budget-friendly option for AI/ML tasks, mainly for new businesses or smaller groups with restricted budgets. Moreover, the availability of a budget-friendly GPU cloud makes it easy for a huge variety of users to use, especially those using AI for the very first time.
Scalability
GPU cloud service providers provide the scalability to easily up or down resources according to project needs. This is useful for a variety of applications like AI/ML, where the requirement for computational power can change during different stages. Instead of using costly assets that can be utilized periodically, organizations can adjust their cloud assets according to their needs. Even if you are running limited models or performing challenging simulations, cloud-based GPU solutions offer the required flexibility to fulfill your demands.
Quicker AI Models Training
Both ML and AI models need robust computing power, particularly at the training stage, where huge datasets are quickly processed to enhance the accuracy of the model. GPUs, along with their proficiency to manage parallel processing applications, outshine in this segment. At the time of utilizing NVIDIA GPU cloud solutions, you simply get complete access to several robust GPUs engineered especially for AI-based tasks. It means cloud servers can decrease training times and provide more productivity.
Decreased Operational Costs
Handling on-site servers consists of maintenance, elevations, and managing all possible hardware downtime. GPU4HOST always takes care of your infrastructure and helps clients to focus mainly on their essential tasks and running algorithms. With the help of cloud assets, AI and ML developers remove the working costs related to hardware management, making sure that their assets are always updated on time and fully improved for the cutting-edge AI frameworks.
Cons of GPU Cloud Servers for AI & ML
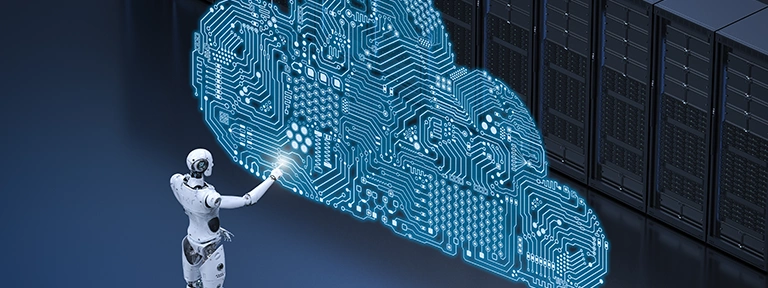
Cost Management
Generally, GPU cloud hosting is more budget-friendly as compared to buying physical resources; the charges can still be included, mainly when performing complex AI or ML tasks. Relying on the size of your available datasets, model difficulty, and the number of GPUs needed, GPU cloud costs can become more noteworthy. For organizations utilizing budget-friendly cloud servers, it’s necessary to successfully monitor utilization and enhance tasks to prevent additional costs.
Data Security & Privacy Concerns
At the time of utilizing GPU cloud solutions, your personal data is kept on third-party servers, which can escalate considerations related to privacy and security. For all those who are managing sensitive data, making sure that their GPU cloud servers adhere to industry guidelines and rules is necessary. While most respected service providers like GPU4HOST apply powerful security practices, businesses must still take several steps to make sure that their data remains safe and protected.
Latency & Network Problems
Since GPU cloud servers depend mainly on an internet connection to use remote assets, network latency can become a major issue, mainly when working with complex datasets. High latency can influence the speed of model training and all other processes, significantly decreasing the complete performance of AI and ML applications. Moreover, bandwidth restrictions can impact data transfer rates among cloud servers and the client’s local infrastructure, resulting in possible interruptions in the case of AI models processing.
Integration Complexity
Including GPU cloud solutions into previous infrastructure can occasionally be difficult, mainly for organizations that already have on-site systems in the proper place. Migration to the cloud needs cautious planning and technical guidance to make sure that AI and ML tasks can smoothly include GPU cloud servers. Relying on the tools and frameworks being utilized, it sometimes takes time to manage workflows to take complete benefit of the cloud GPU assets.
Conclusion
GPU clouds are one of the main resources for AI and ML tasks, providing advantages like flexibility, budget-friendliness, quicker training times, and use of the latest technology, such as the NVIDIA GPU cloud. However, cons like proper cost management, privacy concerns, and integration complexity are required to be cautiously addressed to offer a robust execution of GPU cloud solutions. As GPU4HOST remains to grow, the upcoming time of AI and ML in cloud hosting looks encouraging, offering organizations the computational power they want to remain in the lead in a progressive digital world.
Read More: Best GPU Dedicated Servers